机器学习正对药物发现产生真正的影响——它正使识别最有潜力候选药物的整个过程越来越快。
——Michele Vendruscolo
Machine learning is having a real impact on drug discovery – it’s speeding up the whole process of identifying the most promising candidates
——Michele Vendruscolo
研究人员利用人工智能技术大幅加快了对帕金森治疗方法的探索。
Researchers have used artificial intelligence techniques to massively accelerate the search for Parkinson’s disease treatments.
剑桥大学的研究人员开发运用了一种基于人工智能的方法以识别能够阻止α-突触核蛋白(alpha-synuclein)凝集或凝聚的化合物。α-突触核蛋白是帕金森病的特征蛋白。
The researchers, from the University of Cambridge, designed and used an AI-based strategy to identify compounds that block the clumping, or aggregation, of alpha-synuclein, the protein that characterises Parkinson’s.
该团队利用机器学习技术快速筛选了包含数百万条目的化学库,从而选择出五种高效的化合物进行进一步研究。
The team used machine learning techniques to quickly screen a chemical library containing millions of entries, and identified five highly potent compounds for further investigation.
全球超过六百万人患有帕金森病。到2040年,这一数字预计将翻三倍。目前尚无针对该疾病的改善病情疗法。在大型化学库筛选候选药物的过程非常耗时、昂贵,并且失败率高,而这种筛选必须在对患者进行潜在药测试前就完成。
Parkinson’s affects more than six million people worldwide, with that number projected to triple by 2040. No disease-modifying treatments for the condition are currently available. The process of screening large chemical libraries for drug candidates – which needs to happen well before potential treatments can be tested on patients – is enormously time-consuming and expensive, and often unsuccessful.
利用机器学习,研究人员能够将初始筛查过程加快十倍,并将成本降低一千倍,这可能意味着帕金森氏症的潜在治疗方法可以更快地到达患者端。该结果发表在《自然——化学生物学》期刊上。
Using machine learning, the researchers were able to speed up the initial screening process ten-fold, and reduce the cost by a thousand-fold, which could mean that potential treatments for Parkinson’s reach patients much faster. The results are reported in the journal Nature Chemical Biology.
帕金森病是全球增长最快的神经系统疾病。在英国,如今每37活着的人中就有一人将在一生中被诊断出患有帕金森病。除了运动症状外,帕金森病还可能影响胃肠系统、神经系统、造成睡眠障碍、影响情绪和认知,并可能导致生活质量下降和残疾。
Parkinson’s is the fastest-growing neurological condition worldwide. In the UK, one in 37 people alive today will be diagnosed with Parkinson’s in their lifetime. In addition to motor symptoms, Parkinson’s can also affect the gastrointestinal system, nervous system, sleeping patterns, mood and cognition, and can contribute to a reduced quality of life and significant disability.
蛋白质在细胞工作中非常重要,但当人们患有帕金森病时,这些蛋白质会失控,导致神经细胞死亡。当蛋白质错误折叠时,它们会形成称之为路易体的异常簇积聚在大脑细胞内,阻碍大脑细胞的正常工作。
Proteins are responsible for important cell processes, but when people have Parkinson’s, these proteins go rogue and cause the death of nerve cells. When proteins misfold, they can form abnormal clusters called Lewy bodies, which build up within brain cells stopping them from functioning properly.
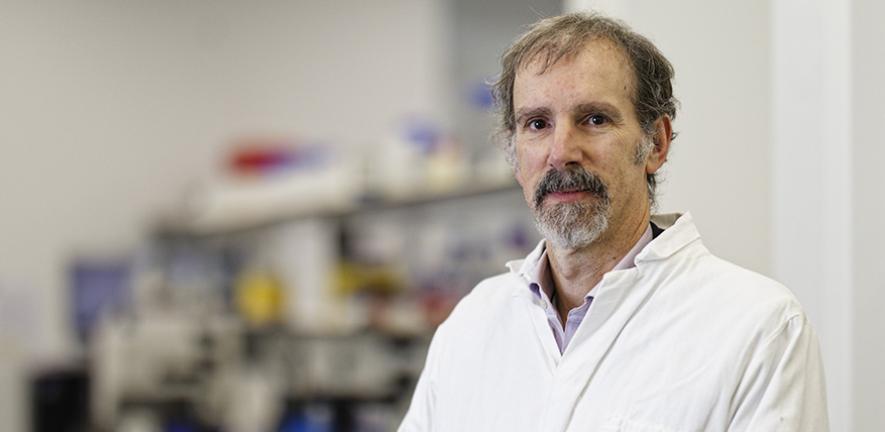
“寻找帕金森病潜在治疗方法的一个方向是找到能够抑制与这个疾病密切相关的α-突触核蛋白聚集的小分子。”领导这项研究的化学系Michele Vendruscolo教授说。“但这是一个极其耗时的过程——仅仅确定一个可进入下一步测试的首要候选药物就可能需要数月甚至数年的时间。”
“One route to search for potential treatments for Parkinson’s requires the identification of small molecules that can inhibit the aggregation of alpha-synuclein, which is a protein closely associated with the disease,” said Professor Michele Vendruscolo from the Yusuf Hamied Department of Chemistry, who led the research. “But this is an extremely time-consuming process – just identifying a lead candidate for further testing can take months or even years.”
虽然目前帕金森病的临床试验正在进行中,但还没有一种改善病情的药物得到批准,这反映出目前我们无法直接以引发该疾病的分子类型为靶点。
While there are currently clinical trials for Parkinson’s currently underway, no disease-modifying drug has been approved, reflecting the inability to directly target the molecular species that cause the disease.
这一问题一直是帕金森病研究的重大障碍,因为目前没有识别正确的分子靶点并与之结合的方法。这种技术上的鸿沟严重阻碍了有效治疗方法的开发。
This has been a major obstacle in Parkinson’s research, because of the lack of methods to identify the correct molecular targets and engage with them. This technological gap has severely hampered the development of effective treatments.
剑桥大学的研究团队开发了一种机器学习方法,该方法对包含数百万种化合物的化学库进行筛选,从而识别能够和淀粉样蛋白聚集体结合并阻止其增殖的小分子。
The Cambridge team developed a machine learning method in which chemical libraries containing millions of compounds are screened to identify small molecules that bind to the amyloid aggregates and block their proliferation.
随后,团队对排名靠前的几种化合物进行了测试,从中选出最具潜力的α-突触核蛋白聚集体抑制剂。再将从这些实验检测中获取的信息以迭代方式反馈到机器学习模型中。这样经过几次迭代后,有明显抑制作用的化合物就能确定了。
A small number of top-ranking compounds were then tested experimentally to select the most potent inhibitors of aggregation. The information gained from these experimental assays was fed back into the machine learning model in an iterative manner, so that after a few iterations, highly potent compounds were identified.
“我们不是通过实验筛选,而是通过计算筛选,”蛋白质错误折叠疾病中心的联合主任Michele Vendruscolo说道。“通过利用我们从机器学习模型的初始筛选中获得的信息,我们能够训练模型识别这些小分子上负责结合的特定区域,然后我们可以重新筛选并找到更有效的分子。”
“Instead of screening experimentally, we screen computationally,” said Vendruscolo, who is co-Director of the Centre for Misfolding Diseases. “By using the knowledge we gained from the initial screening with our machine learning model, we were able to train the model to identify the specific regions on these small molecules responsible for binding, then we can re-screen and find more potent molecules.”
利用这种方法,该团队开发了能与α-突触核蛋白聚集体表面口袋结合的化合物,这些口袋控制了α-突触核蛋白聚集体的指数级增殖。这些化合物比先前报道的化合物更具有数百倍的效力,并且开发成本要低得多。
Using this method, the Cambridge team developed compounds to target pockets on the surfaces of the aggregates, which are responsible for the exponential proliferation of the aggregates themselves. These compounds are hundreds of times more potent, and far cheaper to develop, than previously reported ones.
“机器学习对药物发现真的产生了影响——它正使最有潜力候选药物的整个识别过程越来越快,”Vendruscolo说道。“对我们来说,这意味着我们可以开展多个药物开发项目,而不仅仅是一个。因为时间和成本大幅减少了,一切皆有可能——这是一个激动人心的时刻。”
“Machine learning is having a real impact on drug discovery – it’s speeding up the whole process of identifying the most promising candidates,” said Vendruscolo. “For us, this means we can start work on multiple drug discovery programmes – instead of just one. So much is possible due to the massive reduction in both time and cost – it’s an exciting time.”
该研究于剑桥大学健康化学实验室开展进行,该实验室由英国研究合作投资基金(UKRPIF)支持建立,旨在促进学术研究向临床项目的转化。
The research was conducted in the Chemistry of Health Laboratory in Cambridge, which was established with the support of the UK Research Partnership Investment Fund (UKRPIF) to promote the translation of academic research into clinical programmes.